How can managers and scientists use models to answer questions if they don’t understand the uncertainties of the models? Researchers at Virginia Tech and the Chesapeake Bay Program recently published a paper in Environmental Modeling and Software using a new technique to analyze the uncertainties of three models of the Susquehanna River Watershed. Their results suggest that the models preformed well, but preformed even better as an ensemble.
Zachary Easton, Moges Wagena, and others used a new approach to evaluate three well-vetted models: the Soil and Water Assessment Tool-Variable Source Area model (SWAT-VSA), the standard Soil and Water Assessment Tool (SWAT-ST), and the Chesapeake Bay Program’s Phase 6 Watershed Model (CBP-Model). All three of these models are watershed-scale models used for predicting water quality and flow. The CBP-Model is relatively coarse scale, whereas the SWAT models are finer. They have different structural components that give them different strengths and weaknesses.
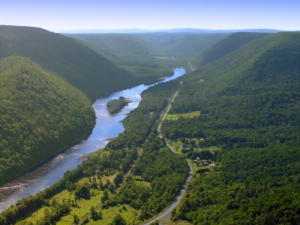
The Susquehanna River running through Pennsylvania. Credit: Nicholas_T/ Via Flickr Creative Commons
In particular, they wanted to understand structural model uncertainties, which they define as the “uncertainty from unknown, simplified, incomplete, or incorrect process descriptions within a model; or simplified mathematical forms or expressions of ecosystem processes in the model.” This type of uncertainty is different than uncertainties around input parameters (the data going into the model) or boundary conditions (the assumptions of the model). In order to understand structural uncertainties, the team used the same input data for all three models so they could directly compare how the models performed against one another. This is the first time these models have been evaluated for structural uncertainties.
It can be difficult to separate this type of uncertainty from the others, but one way researchers have proposed to evaluate structural model uncertainties is by using multi-model ensembles (MMEs), which “combine two or more models in an effort to improve model prediction and objectively evaluate model uncertainty” (Wagena et al 2019). Other models such as Bayesian model averaging (BMA) also could have strengths in understanding structural uncertainties. For this study, the researchers chose to use a new approach, a Bayesian Generalized (Non-) Linear Multilevel Models (BGMM), that specifically looks at structural uncertainties in watershed models.
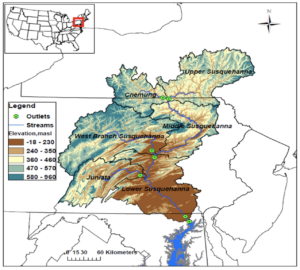
“Location of the Susquehanna River Basin, major subbasins, and elevation features,” from Wagena et al 2019.
In order to understand the uncertainties, they ran both SWAT models, the CBP-model, the BGMM, a BMA, and conducted an evaluation using a straight model average (SMA) MME. They found that the BGMM and the BMA models performed very well, particularly at predicting flow, sediments, and total nitrogen. They stated, “our results show that both Bayesian models performed equivalently, and better than the SMA procedure, particularly for sediment, and substantially better than the individual models, in most cases.” These results are significant because even though the CBP-model, for example, performed better than the SWAT models, the Bayesian models that incorporated the SWAT models and CBP-model performed better than CBP-model or anything else.
Although they didn’t run any management choices in their models, Easton hopes their technique will be able to inform management decisions in the future. “you could use this methodology to evaluate management practices,” he explained, “such as by testing the adequacy of different watershed implementation plans (WIPs), for which this methodology will not only return a prediction of nitrogen load, for instance, but will produce an associated probability of that outcome as well.” Ultimately, this technique will lead to stronger models that can better answer questions about the Bay’s future, as Easton explained. “Understanding uncertainty allows us to create better policies and better management decisions.”